Our lab aims to develop the following systems biology approaches: 1. Multilayered spatiotemporal networks: Complex biological events are commonly regulated by operations of multilayered networks each of which is formed by a different type of regulatory molecules (signaling molecules, transcription factors, micro-RNAs, epigenetic regulators, etc.). For example, the network below shows multiple types of regulators defining multilayered networks for leaf senescence in Arabidopsis (Woo et al., 2013, Journal of Cell Science, 126, 4823-4833). A main research interest in our lab is to develop an experimental and analytical framework to decode multilayered spatiotemporal networks. |
B. Spatial networks: Proteins involve spatial transitions during cellular events. Moreover, the same proteins can be localized in multiple organelles, forming different networks by interacting different sets of molecules in distinct organelles. Combining quantitative and organelle proteomics approaches, spatial networks can be identified. For example, the networks below show a spatial network encoded by nuclear and cytosolic GIGENTEA (GI), regulates circadian rhythm in Arabidopsis (Kim et al., 2013, Developmental Cell, 26, 1−13). |  | By combining genomic (NGS-sequencing), proteomic (mass spectrometry-based proteomics), computational approaches (network-based data integration), we aim to develop an experimental and analytical framework to decode multilayered spatiotemporal networks for leaf aging in Arabidopsis and Rice. | 2. Regulatory motifs or pathways: In complex network models, there are key motifs or pathways that can govern activities of the network models. These motifs or pathways can be identified using various network analysis tools based on diverse centrality measures (e.g. degree and betweeness centralities, as well as random walk-based methods). For example, a transcriptional regulatory pathway to govern down-regulation of the genes involved in oxidative phosphorylation in the presence of a point mutation (A3243G) in the mitochondrial genome (Chae et al., 2013, Science Signaling, 6, 264, doi: 10.1126/scisignal.2003266). 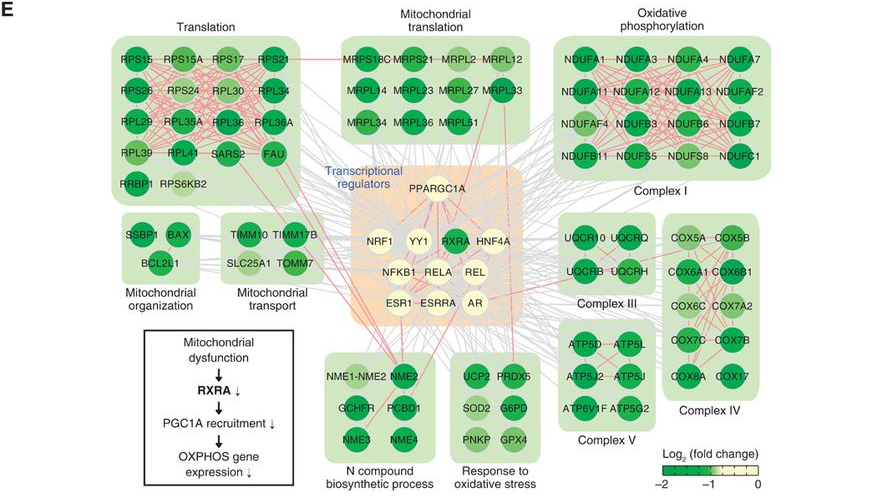 3. Metabolite-protein networks: Metabolites regulate cellular signaling and regulatory networks through their interactions with proteins. Experimental methods involving the conjugation of metabolites to Au-coated magnetic beads coupled with mass spectrometry analysis are being developed to profile the interactors of metabolites involved in a particular metabolic pathway (e.g. ceramide metabolic pathway described in Hannun and Obeid, Nature Reviews Molecular Cell Biology, 9, 139-150). 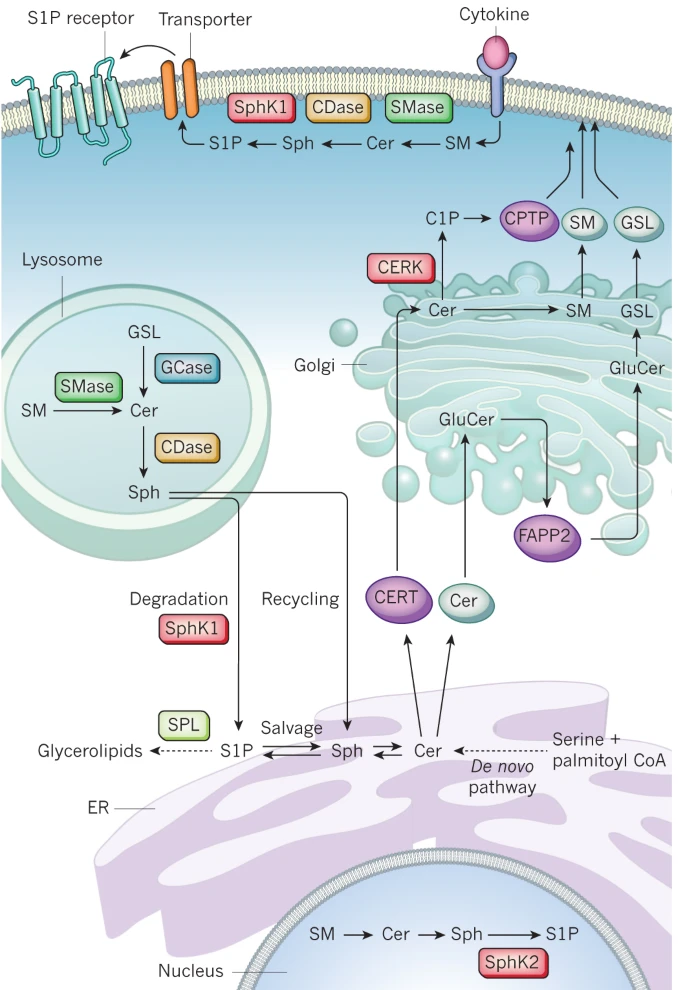 4. Network stochasticity: Various cellular processes have been shown to be stochastic due to the stochastic nature of molecular interactions, leading to stochasticity in biological networks defining the cellular processes. For example, the expression levels of a GFP reporter coupled to an hsp16 promoter in C. elegans varied widely, even among worms that are genetically identical and grown under the same environmental conditions. The lifespan of worms were predicted by fluctuating GFP expression levels, which suggests that gene expression noise plays an important role in determining the longevity (Rea et al., 2005, Nature Genetics, 37, 8, 894−898). After developing multilayered networks for leaf aging in Arabidopsis, we aim to decode the stochasticity of key regulatory motifs or pathways in the multilayered networks using single cell genomic approaches. | 5. Proteomics and informatics: We aim to develop diverse proteomics approaches to monitor posttranslational modifications, localization, and interactomes under different conditions or during cellular events and then to apply them to many biological systems. Also, we have been developing various bioinformatics tools for 1) analyses of genomic, transcriptomic, proteomic, and metabolomic data, 2) integration of multiple types of global datasets (e.g. transcriptomic- proteomic datasets or epigenomic-transcriptomic datasets), 3) construction of static or dynamic networks describing cellular processes differentially regulated during cellular processes, and 4) identification of key regulators that may govern activities of the network models. For example, a framework was developed to decode a network model (see below) for cancer association of tRNA synthetases (ARS) whose functions in cancer are unknown. Also, a bioinformatics framework was developed to decode subnetworks each of which describes cellular processes showing principal differential expression patterns and also to construct time-evolving networks when it was applied to time-course gene expression data. | | | |